Now I'm immediately going to this thought chain:
Maybe such an IQ test could be designed. --> Ah, but then specialized AIs (debatably "all of them" or "most of the non-LLM ones") would just fail equally! --> Maybe that's okay? Like, you don't give a blind kid a typical SAT, you give them a braille SAT or a reader or something (or, often, no accommodation).
The capabilities-in-the-wrong-order point is spot-on.
I could also imagine a thing that's not so similar to IQ in the details, but still measures some kind of generic "information processing/retention/[other activities] capability"... but, as noted, we already can't predict capabilities, so such a measure would need to either solve that or else be less useful than e.g. parameter-count. --> If we classified and properly understood a taxonomy of capabilities, that'd help build such a measure! --> That task, itself, is probably really bloody difficult compared with "some hypothetical tactic that attacks the 'IQ-style fire-alarm' narrative head-on / in a different way".
(A toy demo of "the IQ-style fire alarm won't come" could be a good subtask of "toy demo of misalignment that actually convinces people"... OR it could end up as a "toy demo of capabilities that just pushes people to work more on capabilities", which is obviously bad.)
Most disagreement about AI Safety strategy and regulation stems from our inability to forecast how dangerous future systems will be. This inability means that even the best minds are operating on a vibe when discussing AI, AGI, SuperIntelligence, Godlike-AI and similar endgame scenarios. The trouble is that vibes are hard to operationalize and pin down. We don’t have good processes for systematically debating vibes.
Here, I’ll do my best and try to dissect one such vibe: the implicit belief in the existence of predictable intelligence thresholds that AI will reach.
This implicit belief is at the core of many disagreements, so much so that it leads to massively conflicting views in the wild. For example:
Yoshua Bengio writes an FAQ about Catastrophic Risks from Superhuman AI and Geoffrey Hinton left Google to warn about these risks. Meanwhile, the other Godfather of AI, Yann Lecunn, states that those concerns are overblown because we are “nowhere near Cat-level and Dog-level AI”. This is crazy! In a sane world we should anticipate technical experts to agree on technical matters, not to have completely opposite views predicated on vague notions of the IQ level of models.
People spend a lot of time arguing over AI Takeoff speeds which are difficult to operationalize. Many of these arguments are based on a notion of the general power level of models, rather than considering discrete AI capabilities. Given that the general power level of models is a vibe rather than a concrete fact of reality, it means disagreements revolving around them can’t be resolved.
AGI means 100 different things, from talking virtual assistants in HER to OpenAI talking about “capturing the light cone of all future value in the universe”. The range of possibilities that are seriously considered implies “vibes-based” models, rather than something concrete enough to encourage convergent views.
Recent efforts to mimic Biosafety Levels in AI with a typology define the highest risks of AI as “speculative”. The fact that “speculative” doesn’t outright say “maximally dangerous” or “existentially dangerous” points also to “vibes-based” models. The whole point of Biosafety Levels is to define containment procedures for dangerous research. The most dangerous level should be the most serious and concrete one - the risks so obvious that we should work hard to prevent them from coming into existence. As it currently stands, "speculative" means that we are not actively optimizing to reduce these risks, but are instead waltzing towards them based on the off-chance that things might go fine by themselves.
A major source of confusion in all of the above examples stems from the implicit idea that there is something like an “AI IQ”, and that we can notice that various thresholds are met as it keeps increasing.
People believe that they don’t believe in AI having an IQ, but then they keep acting as if it existed, and condition their theory of change on AI IQ existing. This is a clear example of an alief: an intuition that is in tension with one’s more reasonable beliefs. Here, I will try to make this alief salient, and drill down on why it is wrong. My hope is that after this post, it will become easier to notice whenever the AI IQ vibe surfaces and corrupts thinking. That way, when it does, it can more easily be contested.
Surely, no one believes in AI IQ?
The Vibe, Illustrated
AI IQ is not a belief that is endorsed. If you asked anyone about it, they would tell you that obviously, AI doesn’t have an IQ.
It is indeed a vibe.
However, when I say “it’s a vibe”, it should not be understood as “it is merely a vibe”. Indeed, a major part of our thinking is done through vibes, even in Science. Most of the reasoning scientists rely on for novel research is based on intuitions that would be much too complex to formalize.
Unfortunately, the AI IQ vibe is specifically inadequate to reason about AI progress. And this vibe permeates a lot of existing thinking. Here are two examples.
1. Take Off Speeds, from Paul Christiano
2. ARC’s Safety vs Capabilities Graph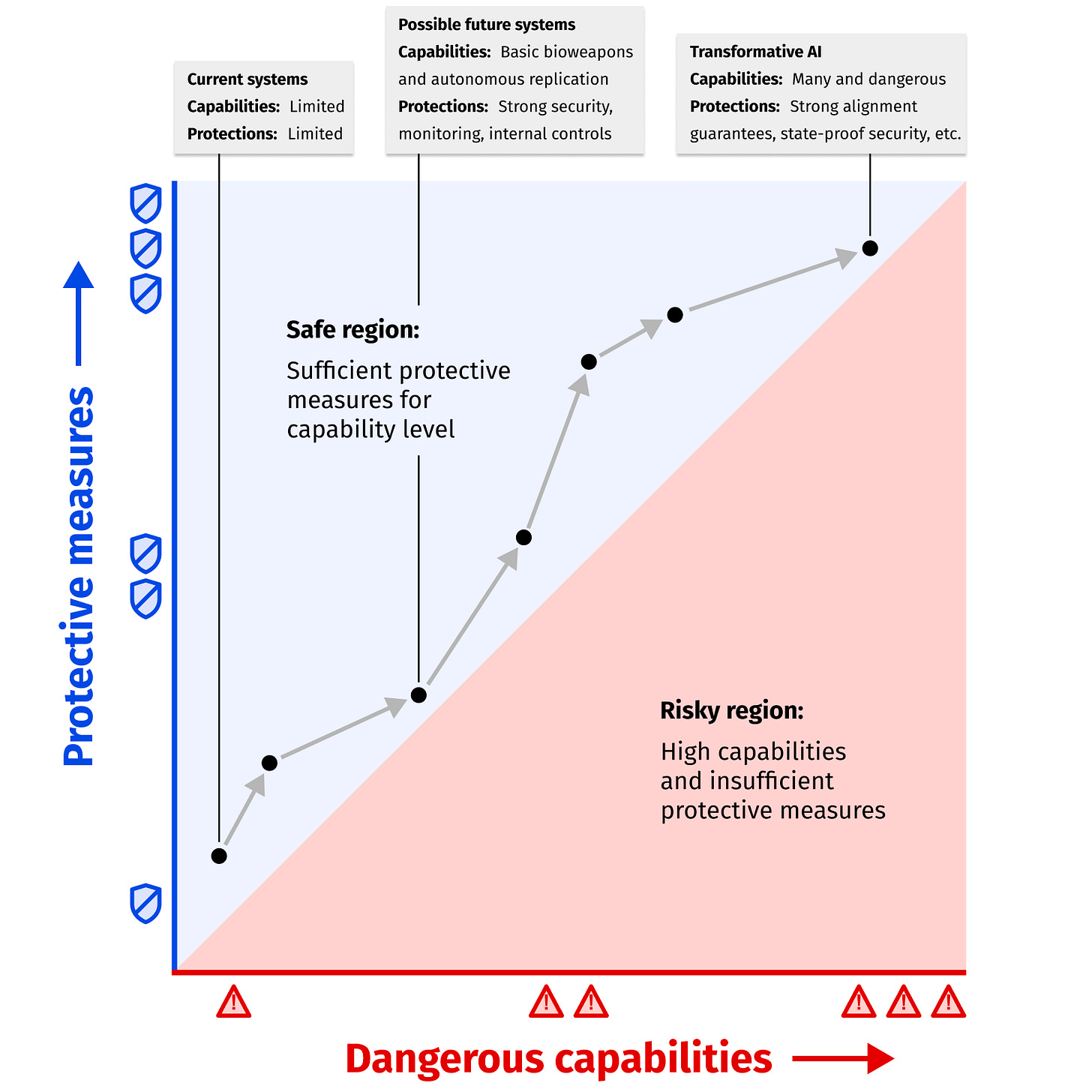
On the first graph, think of what the vertical axis is measuring.
On the second graph, think of what the horizontal axis is describing.
I mean, seriously: what are these axes measuring? The scale of the biggest models? The ability of AI systems to deceive us or be agentic? The number of people killed by AI? GDP growth caused by AI? FLOPs?
If you consider any of these quantities, you will see that they do not capture what the writer meant. This is expected, as vibes are hard to pin down, and AI IQ is one.
House of Cards
Many arguments implicitly rely on this vibe, taking it as obvious. It is crucial to many debates. I have found that once you identify this vibe, you can understand more easily why there can be so much disagreement about extinction risks from AI, and where the disagreement will lie.
My best guess, when talking to people at OpenAI, ARC, Anthropic, DeepMind, and even from what I have read of LeCun: is that the AI IQ vibe is upstream of why they don’t expect AI progress to lead to extinction by default.
There is a common story based on this vibe that goes like:
Sometimes, that frame is used to justify why we should not stop AI progress just yet! The reasoning is that if we paused right now, it would be harder to get warning shots, and these warning shots could have made coordination easier. If you follow that line of reasoning, we should wait until warning shots happen, so that governments are shocked enough to accept a moratorium on AGI progress until we build institutions strong enough to host safe research processes.
This feeling that “we’ll see it coming” if we track AI IQ, which has not (and cannot) be concretely nailed down, allows for some reassurance: there will be a natural point where we will all say “that’s too much”. So we don’t need to do much until then, besides getting more power to use during crunch time.
This also provides reassurance to SuperIntelligence skeptics. AI progresses over time, and you can track it. It will never blow up at once. You can just go with the flow, there won’t be non-recoverable catastrophe, and so you can just react to things as they happen.
Graphical Summary
Here is a tentative graphical summary of the Warning Shots story. It’s a bit cheeky, but hopefully a nice starting point.
You could draw a similar graph for the many other AI IQ based stories.
Unfortunately, there is no AI IQ.
I think you could see it coming from a mile away, but let me state it clearly: there is no AI IQ.
More generally, there is no fire alarm for AGI, but especially not in the form of an AI IQ test showcased to the entire world.
Here is some evidence for there being no such thing.
There is no AI IQ test
No one can agree on where we are on the AI IQ graph. If there was such a thing as AI IQ, you could measure it with a test, and then it would be straightforward to know where we stand. Instead, we are still arguing about dog-level, roughly human-level or junior-programmer-level AI systems.
The closest things that we have to IQ tests for models are benchmarks. But in practice, benchmarks do not mean much. Like, what do you get from the ImageNet or HellaSwag benchmarks beyond “wow, there sure was some progress in the last few years on some test that some team designed”?
If you want more benchmarks, you may consider MMLU, Arithmetic Reasoning, Code Generation or Visual Question Answering.
An alternative would be to check AIs on tests designed for humans. The generic ones like SAT and the ACT, the more specialized ones the LSAT or the bar exam. We could go further and even just administer human IQ tests.
This does not work: these tests have been optimized to measure human skills. And AI systems are alien: they operate very differently from the way people do. Look at how GPT4 performs on various exams, and think about what IQ this would imply in a human being. How would it translate into AI IQ?
The consequence of all of this is the proliferation of rubrics and grading systems, with many papers creating a custom one. They have very poor descriptive power: until you actually look at their data, it’s hard to understand what they purport to measure. And they have even less predictive power: I can’t remember a time where looking at one of these standard benchmarks helped me understand an AI system better.
There is no AI g-factor
Human G-Factor
In humans, IQ tests measure a g-factor (I recommend reading that page, it is quite informative). The idea of a g-factor is that while there might not be a variable or gauge in your brain labeled “intelligence”, we can certainly measure different correlations between people’s skills. While the g-factor is certainly not bulletproof, and the IQ-test even less so, it does offer significant diagnostic value. If we say that someone has an IQ of 150, this tells us something about their ability to learn new information and acquire new skills. While people can sometimes struggle or excel at specific skills, g-factor (as measured by IQ) remains a reliable statistical predictor of many facets of how humans think and learn.
From Wikipedia:
On the first picture, the blue ovals represent the performance at different mental tests, and their intersection with the orange disk represent how much the result of the test can be predicted from the g-factor (itself measured by sampling random tests).
On the second picture, we can see that very different mental tests, like vocabulary and arithmetics, still have a non-trivial pairwise correlation. More plainly: knowing how much better someone is at arithmetic than average lets us make a much better prediction at how good they’ll be at vocabulary, and vice-versa.
It is one of the most replicated results in psychology. It is not just a vibe. We know things that cause g-factor to drop in both the short term and long term: such as stress, sleep deprivation, and malnourishment, and we know that these things result in lower results on the tests and all measures of real-world performance. We know that there is a lot of variation on it between people, and that this variation is unfair. By evaluating someone’s learning skills on a couple of fields at random, you can get a picture of how hard it will be for that person to learn skills from other fields. That picture will be incomplete, but it will give you some non-trivial information.
No AI G-Factor
There is no equivalent in AI. You don’t have well replicated correlations between AI skills, such that from sampling an AI on random tests, you could predict other capabilities. If there was such a thing, then we should be able to predict new capabilities as AIs get better. For instance, “dog level AI” would be able to follow simple orders, but not talk or generate images. Certain benchmarks would indicate certain abilities with some reliability. A measure of a model’s AI IQ would let us know what to expect from it.
Unfortunately, the reality is the complete opposite: AI capabilities have always come in the wrong order!
We initially thought Chess and Logic were the pinnacle of thinking. When this got proven wrong, we moved on to Art and Language. This again was proven wrong. AIs became superhuman at all of these tasks before everyone agreed on us reaching AGI.
We have all seen the ways that image-generation models succeed brilliantly at rendering pictures that easily replicate photographs to a level that even the best painter would struggle with … only to then include a few extra fingers. We have seen LLMs write eloquent paragraphs and rhyming poetry, to only then fail at counting the number of “i” in inconspicuous.
As researchers moved from GPT-2 to GPT-3, they were discovering abilities, often being surprised by them as the model grew. This is not what it looks like to have identified a g-factor!
Straightforward anthropomorphisation mis-predicts even more: given how good SOTA LLMs are at passing exams, you would expect them to be geniuses. Yet, they still fail at consistently following simple orders. No one would have predicted that we would have AIs that are routinely used to write essays and emails, but that the best one would fail at counting the number of “i” in “inconspicuous”.
Nevertheless, we can still see people reaching for the vibe explicitly in DeepMind AGI levels or implicitly in Anthropic's AI Safety Levels.
Finally, capabilities are increasingly happening through fine-tuning, scaffolding, integration with outside resources, and other approaches. These make AI g-factor even less coherent as a concept: the AI system is less and less a single unified piece of software, and now evolves as a result of its interaction with its environment and its developers.
Conclusion: The Vibe is Broken and Gamed
There are no AI IQ tests, and no capabilities predictions were made on the basis of some correlation behind AI capabilities. But the vibe persists! The expectation of a warning shot never dies!
5 years ago, if an oracle told us that in 2023, Hollywood writers would go on strike with the goal of not being replaced by AIs, we would have thought it was a skit. If the AI Safety community was told it was going to happen, everyone would have agreed that this is A Big Thing. Possibly even a famed Warning Shot! Like, this is Sci-Fi level!
Unfortunately, this has not happened. A Vibe is very social, and represents how people around you feel. If you feel that things are normal and progress naturally, you will convey this to others. And if you happen to set the vibe, like Sam Altman spreading AI as eagerly as possible so that people get used to it, then everyone will feel like things are normal, and this will be The Vibe.
Vibes make it easy for the baseline to shift, normalcy to creep, or frogs to be boiled. By making things fuzzy, they make it hard to notice when we were wrong.
And this is what happened. By always pushing the moment where we should Really Care and Start Really Pushing For Things to “later”, both AI developers and the AI Safety community helped deem acceptable all that was and is happening.
Right now, we are at the point where some startup raising hundreds of millions is complaining about some minor EU regulation on LLMs. Imagine a world where private companies built nuclear reactor technology. They kept predicting wrong things about the outcome of their experiments with failures bigger than expected. Then they kept sinking more and more money into bigger and bigger experiments. And finally, they complained about requirements from states to keep continuing their activity (let alone nationalizations!).
The Vibe is completely broken. It is gamed by people acting for the benefit of their own organizations.
This is just not what a surviving, thriving and winning civilization looks like.
Humanity just looks like a frog that is being boiled. On the current path, if it starts reacting to extinction threats, it will be long past the point of no return.
—
In a future post, I will describe a model alternative to the AI IQ vibe, and what it entails regarding curtailing extinction threats from AI.